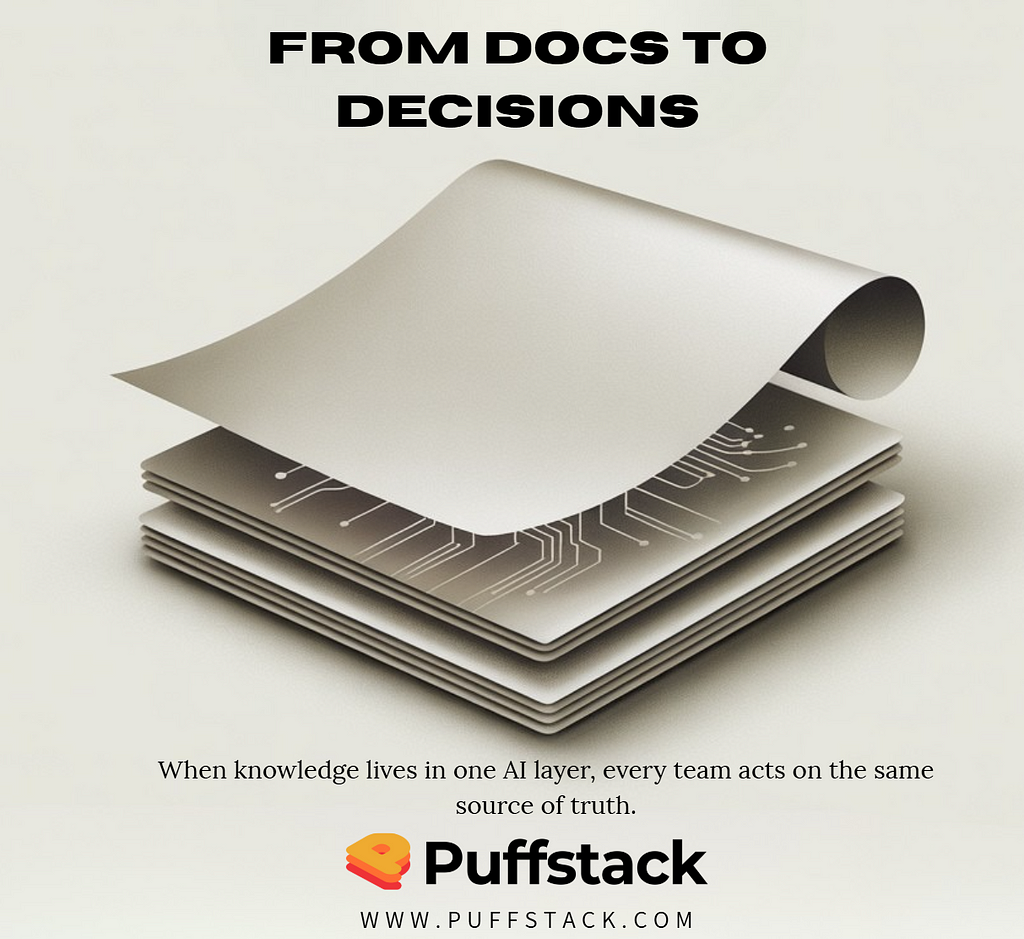
The Real AI Native Strategy
June 30, 2025
Companies often approach AI as another software tool useful but peripheral. They buy AI like they buy accounting software: install it, run it, and expect incremental improvements. But the future belongs to companies that realize AI isn’t just another tool; it’s an entirely new way to structure operations. It’s about building an AI native strategy. Zapier CEO Wade Foster recently captured this paradigm shift perfectly, highlighting that the next generation of companies won’t merely “adopt” AI but will instead be built AI native from the ground up. Being AI native means embedding intelligence deeply into your operational DNA, not just plugging in isolated tools. This distinction matters profoundly for enterprises. According to recent insights from Meta and Alvarez & Marsal, over 70% of high-growth startups integrating AI directly into their core workflows have reported around a 30% reduction in customer acquisition costs. Gartner’s latest data reinforces this, predicting that by 2026, 80% of enterprise applications will come with embedded AI functionality. JPMorgan’s recent CIO survey underscores this point, revealing a sharp pivot toward buying integrated AI capabilities rather than building from scratch, with CIOs shifting significant portions of their budgets toward AI solutions. However, many organizations still mistakenly treat AI as isolated solutions individual chatbots, single purpose analytics engines, and fragmented tools that never fully communicate. These “bolt on” approaches create silos, wasting potential and undermining efficiency. True AI native strategy dismantles these silos by integrating AI deeply within the enterprise fabric, allowing AI to become an active collaborator rather than a passive tool. At Puffstack, our philosophy revolves around building this deep, integrated intelligence. Instead of isolated “agents,” we engineer AI into the connective tissue of enterprise operations. Think of it as wiring intelligence into your workflows. This integration allows AI to execute complex tasks across departments seamlessly like proactively diagnosing issues by synthesizing support tickets, manuals, and historical data, or dynamically generating customized reports from fragmented data sources. Restructuring operations around intelligent systems is the key. For instance, an AI native approach might allow a quality manager to request, “Identify recurring failure patterns in equipment downtime reports,” with integrated intelligence analyzing maintenance logs, sensor readings, and operational data to generate precise, actionable insights immediately. This is transformative, strategic AI in action. Working with Puffstack means adopting this future proof, AI native mindset. Our integrated AI layer goes beyond answering simple queries. It actively orchestrates complex workflows, aligns teams with data driven insights, and continuously learns from organizational interactions to improve institutional knowledge. This approach doesn’t just lower support costs or improve efficiency marginally; it fundamentally reshapes your business processes to be smarter, faster, and inherently scalable. It positions your company not as a consumer of AI, but as a collaborator with AI. The future belongs to AI native enterprises. Those who embrace this strategy early will secure a competitive advantage that late adopters won’t easily overcome. If you’re curious about what an AI native strategy looks like for your enterprise, let’s explore it together. Book an AI integration assessment session no sales pitch, just strategic alignment. Let’s find the opportunities where embedded intelligence can transform your operations, now and in the long term.
Read more